Since AI technologies have been used by many patent applicants who were not familiar with information and communication technologies, the Japan Patent Office (JPO) has had to demonstrate what kinds of inventions are patent eligible and how the specification should be described.
The JPO published five case examples in 2017 and 10 in 2019. However, due to the rapid development of AI technologies and an increased number of applications, the existing examples could not cover certain types of inventions. The JPO updated its examination guidelines by adding case examples to the examination handbooks on March 13 2024, in response to requests by many Japanese patent applicants.
The case examples added in those updates are shown in the below table.
No. | Requirement | Title of invention | Note | Reference |
1 | Inventive step | Automatic answer generator for customer centres | Simple systemisation using generative AI (large language model, or LLM), which replaces human performance | Appendix A-5, Case 37 |
2 | Inventive step | Methods for generating prompt sentences for input into LLMs | Features (prompt generation) in the application of generative AI (LLMs) | Appendix A-5, Case 38 |
3 | Inventive step | Training method for trained models used to adjust the brightness of radiological images | Training method for a trained model to estimate output data from input data | Appendix A-5, Case 39 |
4 | Inventive step | Laser processing apparatus | Systemisation of human performance | Appendix A-5, Case 40 |
5 | Enablement support | Fluorescent luminescent compounds | Invention of things presumed by AI to have a certain function (material informatics) | Appendix A-1, Case 52 |
6 | Support | Image generation methods for teaching data | Method for generating teaching data | Appendix A-1, Case 53 |
7 | Support | Machine learning device for screw tightness quality | Case related to an input-output relationship between several types of data in the teaching data being unclear/clear | Appendix A-1, Case 54 |
8 | Eligibility | Teaching data and image generation methods for teaching data | Teaching data | Appendix A-3, Case 5 |
9 | Eligibility | Trained model for analysing accommodation reputation | Trained models configured as parameter sets | Appendix B-3-2, Case 2-14’ |
10 | Clarity | Trained models for outputting the work to be carried out on anomalies | Trained model of “program” or unknown | Appendix A-1, Case 55 |
Inventive step
Additional case 2
Claim 1 A method of generating a sentence for a prompt, wherein the prompt is generated by a computer for input into a large-scale language model by adding reference information to an input question sentence, wherein the large-scale language model has a maximum number of input characters, and outputs answer sentences in response to a prompt including input question sentences. The method involves generating, by computer, an additional sentence that relates to the question sentence such that the total number of characters, including the number of characters of the question sentence, is within the maximum number of characters based on the input question sentences, and generating, by computer, the prompt by adding the additional sentence as the reference information to the input question sentence. Claim 2 The method of claim 1, wherein generating the additional sentence involves obtaining a plurality of related sentences that relate to the input question sentences, extracting a plurality of keywords that are appropriate for the reference information from the plurality of related sentences, and generating the additional sentence, in which the total number of characters is within the maximum number based on the plurality of keywords. |
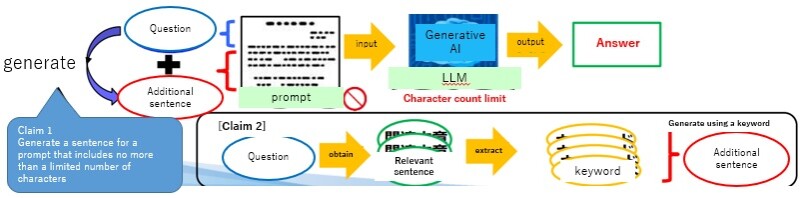
The invention relates to how to generate additional sentences to input into generative AI, such as a large-scale language model with a question sentence. The language model has a maximum number of characters and the additional sentences are generated such that the total number of characters, including the question sentence and the additional sentences, is within the maximum number. The difference with the prior art was underlined in the claims.
According to the JPO, the inventive step of claim 1 is denied, while that of claim 2 is accepted. The JPO understands that the difference in claim 1 is an obvious problem to avoid the amount of information processing, and limiting the number of input characters is a well-known technology. On the other hand, for claim 2, there is no prior art and it has a remarkable effect wherein more appropriate additional sentences can be generated based on relevant keywords.
Since the evaluation of differences from the prior art largely depends on the recognition of the state of art, it is unclear whether this example will improve applicants’ predictability.
Enablement/support requirements
Additional case 5
Claims 1, A fluorescent emissive compound having a luminescence peak wavelength of 540 to 560 nanometres (nm) and a fluorescence lifetime of 5 to 20 microseconds (μs); 2, The fluorescent emissive compound of claim 1, wherein the fluorescent emissive compound is compound A; and 3, The fluorescent emissive compound of claim 1, wherein the fluorescent emissive compound is compound B. |
Specification Fluorescent emissive compounds whose luminescence characteristics with an emission peak wavelength of 540 to 560 nm and a fluorescence lifetime of 5 to 20 μs were not known. Embodiment 1 Using the trained model, the chemical structures of fluorescent emissive compounds with emission peak wavelengths between 540 and 560 nm and emission properties with fluorescence lifetimes between 5 and 20 μs were predicted, and compounds A and B with novel chemical structures were predicted. Embodiment 2 A manufacturing method for compound A is shown and compound A was manufactured using the manufacturing method. The luminescence properties of compound A were measured, and the emission peak wavelength was 545 nm and the fluorescence lifetime was 12 μs. |
Common technical knowledge at the time of filing The predicted results of a trained model cannot substitute for actual experimental results in the technical field of chemistry. |
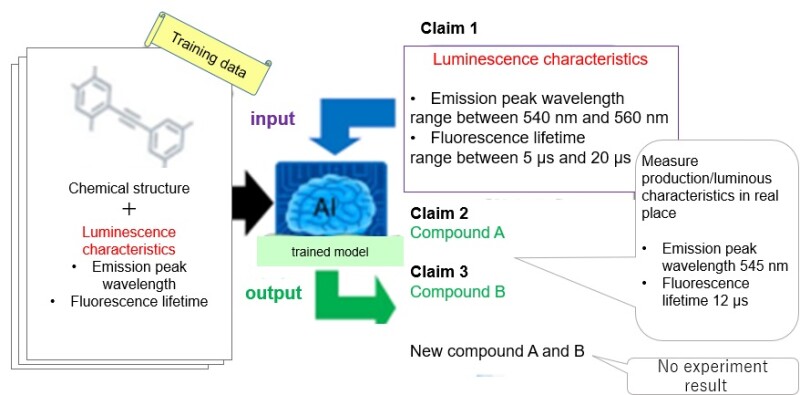
In this example, the trained model predicted two compounds, A and B. According to the JPO, claim 2 for compound A, which was actually produced and the results of which were described in the specification, satisfies the support/enablement requirements. However, claim 3 for compound B, which was not actually produced, and claim 1, which refers to superordinate concepts including components A and B, violate the support/enablement requirements. The JPO explains the reasons as follows:
The predicted results of a trained model cannot substitute for actual experimental results in the technical field of chemistry (as a precondition), and thus it is unclear whether components other than component A have characteristics as predicted by the model (enablement).
The method for producing components other than component A is not disclosed (enablement).
From (1) and (2), the specification does not describe the solution to a problem (i.e., to provide all fluorescent emissive compounds with emission peak wavelengths between 540 and 560 nm, and emission properties with fluorescence lifetimes between 5 and 20 μs). Accordingly, the content of the specification cannot be extended or generalised to the extent of the scope of claim 1 or 3 (support).
Compared with the idea of plausibility, it seems that the JPO is still conservative on this point.
Patent eligibility
Additional case 9
In Japan, an invention is defined as “the creation of a technical idea utilising laws of nature”, and examination for patent eligibility is conducted based on whether a claimed invention corresponds to a “statutory invention”. Patentable subject matters include “products”, “methods”, and “methods to produce products” under Article 2(3) of the Japanese Patent Act. A noteworthy fact is that “programs, etc.” (as intangible objects) are included in “products” under Article 2(4), and the act of working an invention for a program includes the act of providing a patented program, etc. via telecommunication lines. In this context, “programs, etc.” means computer programs and their equivalents, such as data structures.
In the updates in 2019, an eligible category name of “trained model” was clearly indicated.
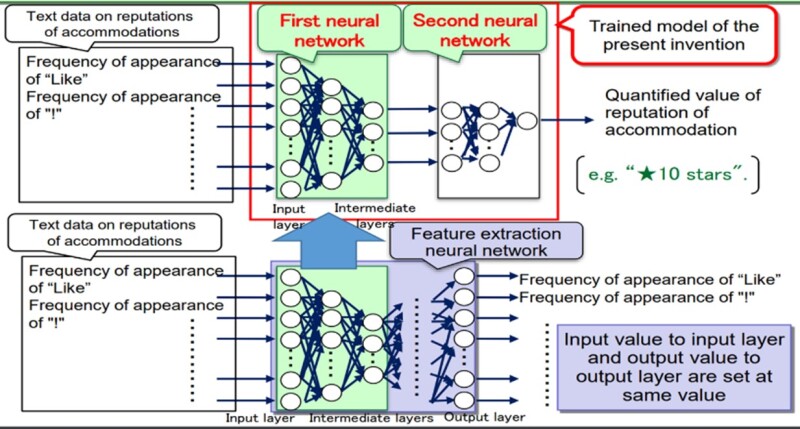
Claim 1 A trained model for causing a computer function to output quantified values of reputations of places of accommodation based on text data on reputations of places of accommodation, wherein the model is composed of a first neural network and a second neural network connected in such a way that the second neural network receives output from the first neural network. The first neural network is composed of an input layer to intermediate layers of a feature extraction neural network in which the number of neurons of at least one intermediate layer is smaller than the number of neurons of the input layer, the number of neurons of the input layer and the number of neurons of the output layer are the same, and weights were trained in such a way that each value input to the input layer and each corresponding value output from the output layer become equal. Weights of the second neural network were trained without changing the weights of the first neural network, and the model causes the computer function to perform a calculation based on the trained weights of the first and second neural networks in response to the appearance frequency of certain words obtained from text data concerning the reputations of places of accommodation input to the input layer of the first neural network and to output the quantified values of reputations of places of accommodation from the output layer of the second neural network. |
There are four important points in claim 1:
The category name of claim 1 is a “trained model”;
The structure of the neural network is recited in detail (which is different from traditional program claims);
The purpose of use (i.e., to output the quantified values of reputations of places of accommodation) is specified; and
The trained model is designed to function on a computer.
In particular, points (3) and (4) are necessary to satisfy patent eligibility in Japan. Specifically, according to the examination guidelines, any information processing by software must be concretely realised by hardware resources. In this context, “concretely” means specific information processing is conducted, depending on the “purpose of use”, through the collaboration of software and hardware.
In contrast to the above case, the JPO added the following example, which does not satisfy patent eligibility under the updates in 2024.
Claim 1 A trained model to output quantified values of reputations of places of accommodation based on text data on the reputations of places of accommodation, wherein the model is a parameter set composed of trained weighted coefficients of a first neural network and trained weighted coefficients of a second neural network connected in such a way that the second neural network receives output from the first neural network. The first neural network is composed of an input layer to intermediate layers of a feature extraction neural network in which the number of neurons of at least one intermediate layer is smaller than the number of neurons of the input layer, the number of neurons of the input layer and the number of neurons of the output layer are the same, and the weights were trained in such a way that each value input to the input layer and each corresponding value output from the output layer become equal. The weights of the second neural network were trained without changing the weights of the first neural network, and the model performs a calculation based on the trained weights in the first and second neural networks in response to the appearance frequency of certain words obtained from text data on the reputations of places of accommodations input to the input layer of the first neural network and to output the quantified values of reputations of places of accommodations from the output layer of the second neural network. |
The difference from the previous example is that a computer (as a piece of hardware) is not recited and the trained model is a parameter set. Accordingly, collaboration between hardware and software is not achieved and the trained model is deemed to be a mere presentation of information (which does not correspond to an “invention”, because it is not a “technical idea” in view of the definition of invention).
From the two examples above, it can be understood that the JPO considers a trained model to be protected as a combination of a “neural network” and a “parameter set” for a neural network that performs actions on a computer.
Clarity
Additional case 10
Claim 1 A trained model for estimating work to be performed in response to an abnormality occurring in a copier, wherein the parameters are trained by using training data in which an error code indicating ‘A’, error location information indicating ‘B’, and label information indicating a content of work performed by a maintenance person for the error. The trained model receives an error code indicating ‘A’ and location information indicating ‘B’ as an input, and estimates the content of work based on the parameters in response to the input error code and the location information. |
Specification The trained model in the present invention may be a program module that is a portion of AI software. The trained model may be stored in the memory if the trained model is a program module. |
The subject matter of claim 1 is also a trained model that is patentable subject matter as described with respect to patent eligibility. In Japan, the scope of an invention must be interpreted based on claims, except in certain circumstances. In this example, there is no recitation where the trained model in claim 1 causes a computer to perform a plurality of functions. Therefore, the description of the specification will be referred to.
The specification describes that “[t]he trained model in the present invention may be a program module, which is a portion of artificial intelligence software, and the trained model being a computer is not necessary.” From the recitation of claim 1 and common technical knowledge, it is not clear that the trained model in claim 1 is a program, and thus it is not clear that the invention is an invention of a product. To overcome the rejection, claim 1 should be amended to recite a computer or the specification should be amended to clearly recite that the trained model is a computer.
Summary of the JPO’s updated examination guidelines on AI-related inventions
In the update in 2024, 10 case examples were added to the examination handbook, for inventive step, support/enablement requirements, patent eligibility, and clarity.
For inventive step, the basic idea is the same as the existing framework, and there are not many important points.
For the support/enablement requirements, an example about chemical components predicted by AI was added. The example was presented under a conservative precondition (i.e., “predicted results of a trained model cannot substitute for actual experimental results in the technical field of chemistry”). The idea of “plausibility” of results by AI is not considered at this time.
For patent eligibility and clarity, some examples of eligibility of a “trained model” were provided. A trained model being a program was emphasised in the examples. The trained model being an eligible category was radical in the update in 2019, but the update this year was rather restrictive.
As a whole, although the 2024 update did not present a unique idea, it is, rather, a supplement to existing examples.
Reference
JPO Additional Case Examples of AI-Related Inventions (Japanese only)